Since 2022, CISESS has funded early-career scientists from within the center with the goals of promoting the development of innovative ideas and identifying emerging topics within the purview of the center’s research objectives. While four projects have been funded for the last four years, this year, five Seed Grants have been awarded. Read on to learn about the work of the seed grant recipients!
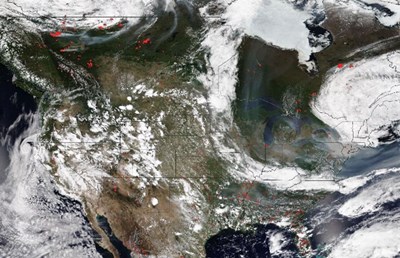
Wildfire Smoke Early Warning: Using Earth Observations to Improve Health Interventions
Evan Ellicott
Associate Research Professor, UMD Department of Geographical Sciences
Wildfires in the U.S. and Canada have become larger and more intense, often displaying extreme behavior. Coupled with increased temperatures and drought, North America has witnessed the emergence of “megafires” (>40,500 ha). The Smokehouse fire in Texas last year (2024) burned an astonishing 1.1 million acres while 8 of the largest fires in California history have occurred since 2017. Colorado has seen the 20 largest fires in state history occur since 2001 with nine of these since 2018. In 2023, Canada witnessed over 17 million hectares burn, approximately eight times the 40-year average (1983-2022). The Canadian wildfires transported smoke to the central and eastern portions of the U.S. causing dangerous levels of air pollution while highlighting the reach and impact wildfires can have beyond their proximate location (see Figure). The team’s research, recently published in the Journal of American Medical Association (JAMA), demonstrated significant increase in ambulatory clinic, inpatient or emergency clinical encounters for cardiopulmonary disease in Maryland during June 2023 when wildfire smoke impact was greatest.
This CISESS Seed Grant Project is developing a wildfire smoke early-warning system using Earth observations, modelled data, and ground measurements to enable timely deployment of clinical support resources to high-risk areas in Maryland. Such a system would have clear benefits to mitigate health care impacts as a result of wildfire smoke events. The alert system will be developed in partnership between the University of Maryland College Park, University of Maryland School of Medicine and University of Maryland Institute for Health Computing (IHC). The team is also working with the Renal Research Institute and the Fresenius Kidney Care (FKC) dialysis clinics. The system architecture will allow transfer of knowledge (code, data sources, etc.) to other regional, state, or municipal systems. The expected science would demonstrate the assimilation of various ground and remotely sensed data for a targeted cause and audience and highlight the effectiveness of Research-to-Operations (R2O).
The team is currently standing up a server to host GeoServer, ingest wildfire activity, including locations, size, and intensity (as estimated from satellite fire radiative power). They will also be ingesting High-Resolution Rapid Refresh Smoke model runs and performing our own modelled smoke trajectories using the Hybrid Single-Particle Lagrangian Integrated Trajectory (HYSPLIT) model. The web dashboard will offer real-time situational awareness of fire and smoke activity in North America and will allow users to subscribe to receive notifications via text or email when a smoke event is imminent (~ 2 days out).
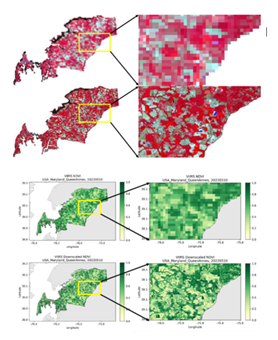
Developing High Spatiotemporal Resolution Vegetation Datasets from Multi-Sensor Satellite Observations for Enhanced Ecological and Agricultural Monitoring
Yuan Zhou
Assistant Research Scientist, UMD/ESSIC/CISESS
Satellite-based vegetation datasets such as Vegetation Indices (VI) and Green Vegetation Fraction (GVF) are essential for monitoring vegetation health, understanding ecological changes, and supporting precision agriculture. However, existing products face trade-offs between spatial and temporal resolution. Coarse-resolution sensors like VIIRS offer frequent global coverage but lack fine detail, while high-resolution satellites such as Sentinel-2 and Landsat provide detailed imagery but with infrequent revisits. This limits their utility for near-real-time agricultural and ecological monitoring, particularly during critical growth periods or rapidly changing environmental conditions. Addressing these limitations is crucial for enhancing food security, supporting sustainable agriculture, and monitoring ecosystem dynamics.
This CISESS Seed Grant Project aims to overcome the spatial-temporal trade-offs by integrating multi-sensor satellite data to develop high spatiotemporal resolution vegetation datasets. Key objectives include: 1) Designing a hybrid framework that combines coarse-resolution VIIRS data with high-resolution Sentinel-2 and Landsat imagery. 2) Pre-calibrating an offline look-up table (LUT) to eliminate dependency on real-time high-resolution data, ensuring efficiency and scalability. 3) Validating the framework using ground measurements for accuracy across diverse landscapes. 4) Producing daily vegetation datasets at 10–30m resolution to support precision agriculture and ecological monitoring. 5) Preparing the framework for operational deployment within NOAA systems to provide consistent, high-quality vegetation products.
The project will deliver: 1) Daily high-resolution vegetation datasets (10–30m). 2) A pre-calibrated LUT for seamless integration of satellite observations. 3) A framework ready for operational deployment, aligning with NOAA’s mission to deliver actionable Earth observation data. A preliminary effort has been conducted to test the feasibility of the proposed method. The Figure above shows a case study in Queen Ann’s county, Maryland, dominated by cropland. The integrated image and the Normalized Difference Vegetation Index (NDVI) map reveal significantly enhanced spatial details compared to their counterparts at the original 1km resolution.
With the successful completion of this project, these datasets will enhance precision agriculture, contribute to sustainable resource management, and improve resilience to environmental challenges, offering tangible benefits to ecological and agricultural stakeholders.
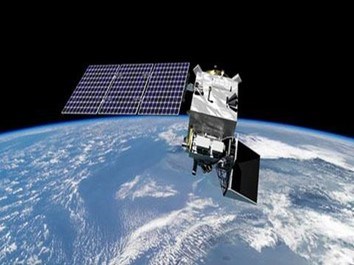
Machine Learning-based Hyperspectral Sensor Data Retrieval at the CISESS Remote Sensing Laboratory
Xi Shao
Research Scientist, UMD/CISESS/ESSIC
The advancements in Earth observation and environmental science are driving the need for remote sensing instruments with higher spatial, spectral, and temporal resolutions. . Hyperspectral imaging (HIS) is a process that divides light into many narrow, contiguous spectral bands across the electromagnetic (EM) spectrum, typically between visible and infrared wavelengths. Different features on Earth’s surface reflect, absorb, scatter and emit light at specific wavelengths, which creates a spectral “fingerprint,” which hyperspectral imagers are able to identify. HSI has become essential for identifying atmospheric constituents, and monitoring greenhouse gases, land surface changes, air quality and marine ecosystem. Both Low Earth Orbit (LEO) and Geostationary (GEO) satellites increasingly utilize hyperspectral sensors. NASA’s Plankton, Aerosol, Cloud, ocean Ecosystem (PACE) Ocean Color Instrument (OCI)was launched in January 2024 and delivers hyperspectral data with over 200 bands, providing insights into seawater composition.
This CISESS Seed Grant Project will expand the work of the student-oriented CISESS Remote Sensing Laboratory by performing field experiments with lab hyperspectral radiometerand developing Machine Learning (ML)-based satellite hyperspectral sensor data retrieval capability. The project will be led by ESSIC/CISESS Scientist Xi Shao, along with Wenhui Wang and Tung-Chang Liu. The seed grant project aims to leverage PACE OCI hyperspectral data to develop ML-based retrieval algorithms tailored for hyperspectral missions. Both supervised and unsupervised ML models will be developed to analyze PACE OCI hyperspectral data for ocean composition classification and algae monitoring. Convolutional Neural Networks (CNNs) are particularly well-suited for the classification due to their ability to capture spatial-spectral patterns. AVariational Autoencoder (VAE) CNN model will be developed to enable unsupervised learning to efficiently encode hyperspectral data and extract spectral features and fractional abundances. The project will train intern students to collect hyperspectral radiometer measurements from field and lab experiments and characterize water sample spectra (Fig. 1 a-c). Techniques such as chlorophyll-a and harmful algae bloom indices (Fig. 1d-g) will be used to characterize water constituent variations. The Remote Sensing Laboratory activities will foster a broader scope of collaboration and engagement between the UMD/CISESS team and the NOAA sensor calibration team. The insights gained from this project will be instrumental in preparing for future hyperspectral imaging sensors.
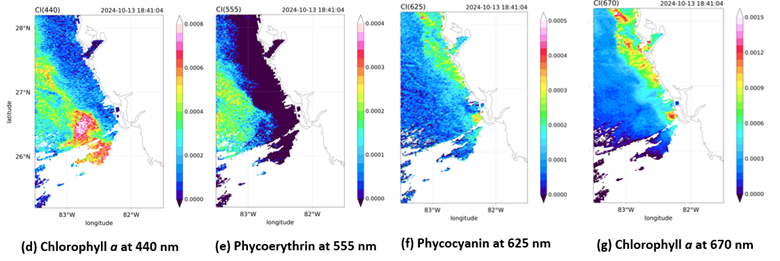
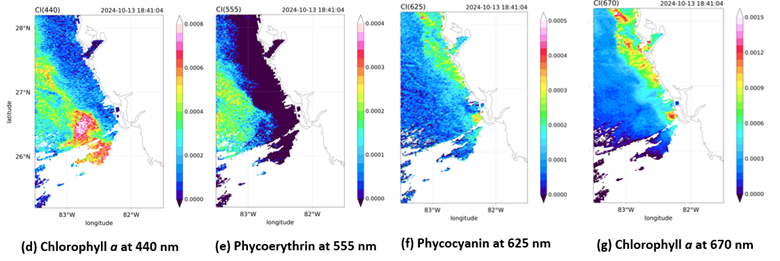
![GPM Dual-frequency Precipitation Radar (DPR) Latent Heat (LH) profile for the period Apr 2014 – Oct 2023. Shownis mean LH profile (K h-1) at 500-meter vertical resolution for precipitating DPR pixels, as a function of DPR [ © ] convective volume fraction (VF) over collocated GMI Field of View. VF value of 0.0 indicates fully stratiform field of view. VF value of 1.0 indicates fully convective field of view.](https://essic.umd.edu/wp-content/uploads/2025/05/Latent_Heat_Profile_and_Thumbnail.jpeg)
Retrieving Latent Heat from Passive Microwave Satellite Observations
Veljko Petkovic
Associate Research Scientist, UMD/CISESS/ESSIC
This CISESS Seed Grant Project seeks to build a robust link between top-of-the-atmosphere microwave radiance and vertical properties of cloud systems. Specifically, the study attempts to demonstrate the feasibility of retrieving the latent heating profile of the atmospheric column using passive microwave satellite observations. It is intended to back a new retrieval development and improve understanding of variability in cloud morphology at a range of spatial and temporal scales. If successful, the new satellite retrieval will support the fundamental science of NOAA’s satellite programs by promoting a) unique cloud system analyses and new insights into cloud processes, b) potential for advances in numerical prediction, and c) a better understanding of energy and water budgets at global and regional scales.
Existing radar- and radiometer-based latent heating products, combined with modeled data and advanced statistical techniques, will capture relevant relationships between passive microwave observations and latent heat distributions. Sensors of specific interest are the GCOM/GOSAT Advanced Microwave Scanning Radiometer (AMSR) series and GPM Dual-frequency Precipitation Radar (DPR). Ultimately, the work is expected to pave a road towards complementing the existing satellite data records with valuable, currently-missing, information significant to Earth’s atmosphere’s radiative balance and hydrology cycle.
Advancements made in deep learning over the past several years, combining physical process-based research with automated data-driven analysis, now enable efficient identification of the links between cloud patterns and their radiometric properties from passive microwave observations proposed here. Therefore, the present study proposes the use of Quantile Regression Neural Networks and similar models to build links between the fields of microwave radiances observed at the top of the atmosphere and radar-observed latent heat profiles. To achieve this, radar-based latent heat profiles observed during a 9-year period (see Figure above) will be collocated with passive microwave observations. These collocations will support a neural network training designed to link the vertical profile of (radar-based) latent heating and passive microwave brightness temperatures.
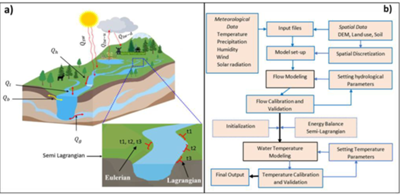
Developing a Basic Model Interface (BMI) for Stream Water Temperature to Enhance Water Quality Predictions in NOAA's Next Generation Water Resources Modeling Framework
Nigus Demelash Melaku
Assistant Research Scientist, UMD/CISESS/ESSIC
Water is vital for life and is intricately connected to environmental conservation, sustainable development, and global stability. While the quantity of water is crucial, its quality is equally important. Water quality serves as a fundamental indicator of environmental integrity, and its preservation is critical for sustaining ecosystem functionality, safeguarding public health, and supporting economic activities. In the context of intensifying urbanization and expanding agricultural practices, the ability to accurately predict stream water temperature has emerged as a pivotal aspect of water quality assessment. Stream temperature significantly impacts aquatic ecosystem dynamics, influencing species composition and biodiversity, altering gas solubility (e.g., oxygen), modulating biogeochemical reaction rates, and regulating the metabolic processes of aquatic biota.
Building on prior findings in developing an advanced stream water temperature model, this project seeks to integrate the team’s semi-Lagrangian formulation into NOAA’s Next Generation Water Resources Modeling Framework (NextGen). The goal is to improve predictions of water quality dynamics across U.S. river basins and provide actionable insights for water resource managers.
The increasing impacts of land use change on aquatic ecosystems such as higher water temperatures, thermal pollution, and shifting species distributions underscore the need for advanced, accurate tools to monitor and manage stream water quality. This approach will enhance the understanding of these challenges and provide real-time insights into stream temperature dynamics, allowing for better decision-making under fluctuating weather conditions.
This initiative aligns with NOAA’s own support of management of water quality risks in environments impacted by flooding and prolonged inundation, as outlined in the 2023 Water Availability, Quality, and Risk challenges.
This CISESS Seed Grant Project will develop a Basic Model Interface (BMI) for stream water temperature modules, enabling seamless integration with NOAA’s NextGen framework. Key objectives include:
- Interface Development: Develop BMI that enables integration of the stream water temperature model with the NextGen platform.
- Testing and Validation: Conduct rigorous testing and validation of the BMI to ensure model accuracy and reliability.
- Real-Time Insights: Provide real-time predictions of stream temperature dynamics, offering valuable data to inform water resource management.
This initiative represents a critical step toward advancing water quality prediction tools and supporting the management of aquatic ecosystems in an era of constant change. By improving our ability to predict stream water temperature dynamics, we hope to contribute to more informed decision-making and more effective water resource management strategies.