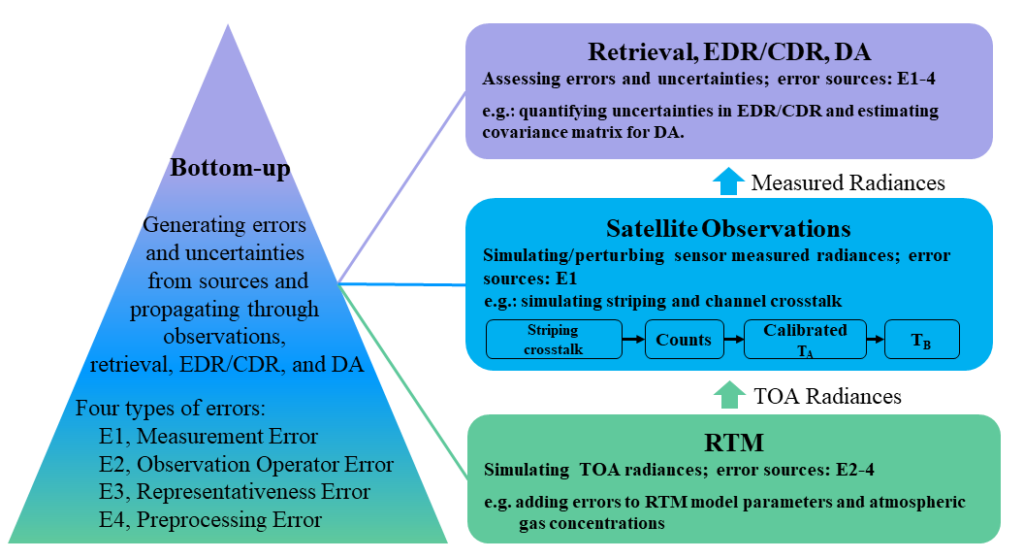
Satellite observations are vital for weather forecasts, climate monitoring, and environmental studies. In recent years, there has been a concerted effort to develop methods for quantifying and representing errors associated with satellite observations. ESSIC scientist John Xun Yang has led a team of scientists in the creation of an error inventory simulator, the Satellite Error Representation and Realization (SatERR). This work is published in AMS flagship journal, Bulletin of the American Meteorological Society.
SatERR is designed to simulate a wide spectrum of observation errors, from instrument measurement to model assimilation errors. To be more specific, SatERR encompasses four distinct categories of satellite observation errors:
- Measurement Errors: These errors stem from instrument imperfections, including biases and noise in the radiance measurements.
- Observation Operator Errors: This category accounts for inaccuracies resulting from deficiencies in the forward model, such as those encountered in radiative transfer models (RTMs) used during satellite observation assimilation.
- Representativeness Errors: These errors arise from unresolved scales and processes, often tied to model parameterization and scale resolution.
- Preprocessing Errors: This category pertains to errors incurred during the data prescreening process.
SatERR’s comprehensive framework allows for the detailed analysis and mitigation of these errors, enhancing the accuracy and reliability of satellite observations for crucial applications in weather, climate, and environmental sciences.
Yang is an Associate Research Scientist at ESSIC/CISESS. His research areas include Earth remote sensing, microwave radiometry, hardware development, calibration, and retrievals. He has been involved with a number of NASA/NOAA satellite missions, including the Aquarius, GPM, CYGNSS, JPSS, and TROPICS. He is a senior member of IEEE and an associate editor of IEEE Journal of Selected Topics in Applied Earth Observations and Remote Sensing (JSTARS).
Yang’s coauthors include ESSIC/CISESS scientists Cheng Da, Christopher Grassotti, Ralph Ferraro, Huan Meng, Jifu Yin and Veljko Petkovic, as well as researchers from NOAA, Massachusetts Institute of Technology (MIT), University of North Carolina, Wilmington, European Organisation for the Exploitation of Meteorological Satellites, Jet Propulsion Laboratory, NASA Goddard Space Flight Center, Ball Aerospace and Technology Corporation, and University of Wisconsin–Madison.
To access the article, click here: “SatERR: A Community Error Inventory for Satellite Microwave Observation Error Representation and Uncertainty Quantification”.