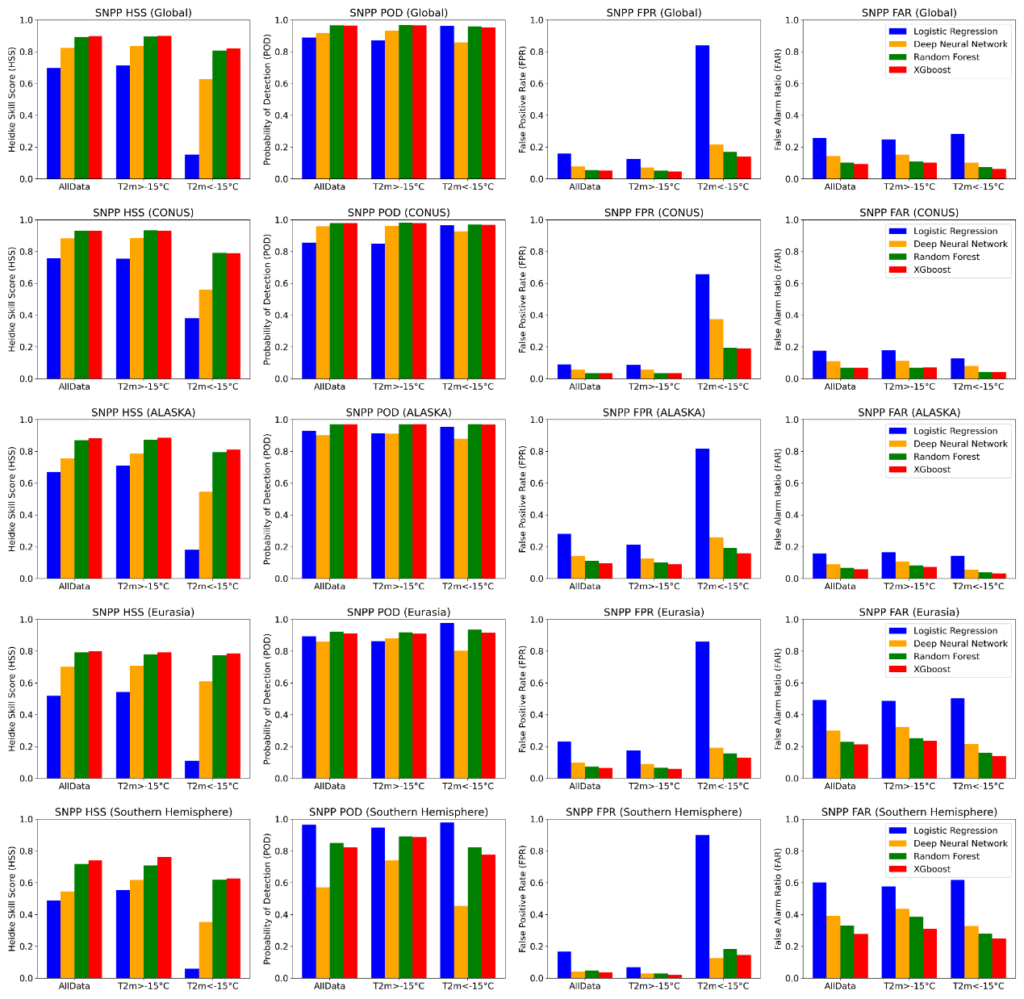
ESSIC/CISESS scientists Yongzhen Fan and Huan Meng have recently developed a new machine learning snowfall detection (SD) algorithm, based on eXtreme Gradient Boosting (XGB). The algorithm was developed for the Advanced Technology Microwave Sounder (ATMS) onboard NPP and NOAA-20 as well as the MHS/AMSU-A onboard Metop-A, Metop-B, Metop-C and NOAA-19.
Four feature engineering algorithms were developed and applied to the global training dataset, which consists of the ground truth from NOAA Integrated Surface Database (ISD) and collocated satellite observations and GFS model analyses, to identify the 30 most important features for detecting snowfall. Hyper-parameter of the XGB model, such as learning rate, number of trees, maximum depth, etc. was tuned to ensure best performance.
The XGB SD algorithms were validated globally and show significantly improved performance compared to the previously developed logistic regression (LR) and Deep Neural Network (DNN) SD algorithm, especially in cold regions and southern hemisphere where training data was not sufficient. The XGB model also slightly outperformed the previously trained Random Forest (RF) model. Fig. 1 shows the validation results of the S-NPP XGB SD model with its comparisons with some other SD models for different geographic regions. Other satellites have comparable performance. A notable advantage of the XGB model over the RF model is that the former has much shallower tree structure than the latter. It makes the XGB transition to operational feasible because the coding of this model is orders of magnitude simpler than that of the RF model. To integrate the XGB SD model in the SFR algorithm, a generalized tree-based data structure and classification algorithm was designed and implemented, which also supports other decision tree and RF based machine learning models.
Fan received the B.S. degrees in electronic science from Xi’an University of Science and Technology, Xi’an, Shaanxi, China in 2004 and the Ph.D. degree in Physics from Stevens Institute of Technology, Hoboken, NJ, USA in 2016. He is currently an Associate Research Scientist with the Earth System Science Interdisciplinary Center (ESSIC) and the Cooperative Institute for Satellite Earth System Studies (CISESS)-Maryland, University of Maryland (UMD), College Park, MD, USA. His research interests include radiative transfer theory, machine learning, satellite remote sensing of ocean color, aerosols and snowfall.
Meng is a physical scientist with NOAA/NESDIS Center for Satellite Applications and Research, Satellite Climate Studies Branch, and a Visiting Research Scientist at ESSIC. She received a MS in Physical Oceanography from Florida State University in 1993, and a PhD in Hydrology from Colorado State University in 2004. She has been working in the field of satellite remote sensing since 1999. Her current research focuses on snowfall retrieval using passive microwave measurements from polar-orbiting satellites.