ESSIC/CISESS scientist Chris Grassotti along with CIRA and NOAA researchers Shuyan Liu and Quanhua (Mark) Liu, recently published a paper in the IEEE Journal of Selected Topics in Applied Earth Observations and Remote Sensing titled “Warm-Season Microwave Integrated Retrieval System (MiRS) Precipitation Improvement Using Machine Learning Methods”.
The study compared the performance of five selected machine learning models regarding precipitation climatology during the warm season in 2022 and 2023 over the Continental U.S. Input features included retrieved products from the Microwave Integrated Retrieval System (MiRS) based on NOAA-20 ATMS data. Among the models, three used a U-Net architecture and two used a Deep Neural Network (DNN) architecture. The U-Net models all significantly outperformed DNN models for the evaluated metrics. While the DNN architecture can only learn from local inputs, the U-Net also has the capability to learn from neighborhood spatial patterns. As such, the DNN overcorrected the precipitation amounts that MiRS had overestimated, leading to net underestimation, but also failed to improve the overall performance relative to the original MiRS estimates. The U-Net not only corrected MiRS overestimation in the central U.S., but also improved the MiRS dry bias over the Southeast.
Of the five experiments, that which used the MiRS retrieved column-integrated hydrometeors of graupel water path, rain water path, cloud liquid water, total precipitable water and geolocation information demonstrated the best performance, improving the MiRS spatial correlation coefficient from 0.75 to 0.89 and reducing the mean bias percentage from 11.95% to -6.33% for 2022 accumulated precipitation. This suggests that applying an appropriate architecture and input features provides an opportunity to determine more accurate physical and statistical relationships which can include spatial and regional dependence, leading to improved microwave-based precipitation estimates.
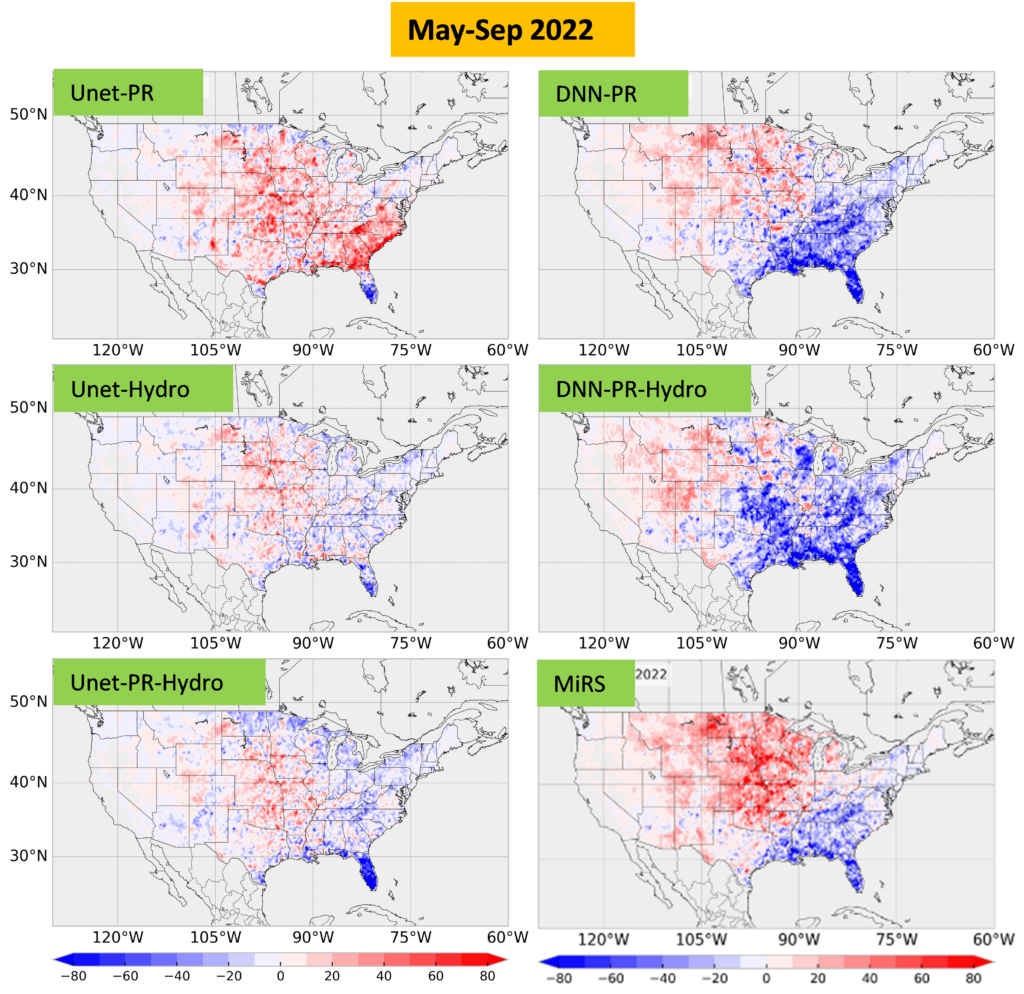
Christopher Grassotti received a B.S. degree in earth and space science from the State University of New York at Stony Brook, Stony Brook, NY, USA, in 1982, tan M.S. degree in meteorology from the University of Wisconsin-Madison, Madison, WI, USA, in 1986, and an M.S. degree in viticulture and enology from AgroMontpellier, Montpellier, France, in 2007. From 1986 to 1991 and again from 1993 to 2005, he was a Research Associate, Senior Research Associate and Staff Scientist with Atmospheric and Environmental Research, Inc., Lexington, MA, USA. From 1991 to 1993, he was with the Atmospheric Environmental Service, Environment Canada, Dorval, QC, Canada. Since 2008, he has been with the National Oceanic and Atmospheric Administration, NOAA Center for Satellite Applications and Research, National Environmental Satellite, Data, and Information Service, College Park, MD, USA. He has been a Senior Faculty Specialist with ESSIC/CISESS since 2015.
To access the work, click here: “Warm-Season Microwave Integrated Retrieval System (MiRS) Precipitation Improvement Using Machine Learning Methods”.