ESSIC/CISESS scientists Veljko Petković, Ralph Ferraro, Malarvizhi Arulraj, and CISESS Summer Intern Vesta Gorooh (UCI PhD student) have a new article in the November issue of the Journal of Hydrometeorology. The article describes the use of machine learning techniques to improve the retrieval of surface precipitation from passive meteorological sensors aboard geosynchronous Earth-orbiting (GEO) and low Earth-orbiting (LEO) satellites.
The researchers used a U-Net–like architecture to map instantaneous, summertime surface precipitation intensity at a spatial resolution of 2 km. As inputs, they used the Global Precipitation Measurement (GPM) Microwave Imager (GMI) and the GOES-R Advanced Baseline Imager (ABI) along with total precipitable water and 2-m temperature from the Global Forecast System (GFS) model. They found that the U-Net algorithm could capture fine-scale patterns and intensity of surface precipitation at high spatial resolution for both stratiform and convective precipitation regimes. The evaluations also revealed the potential of extracting relevant, high spatial features over complex surface types such as mountainous regions and coastlines.
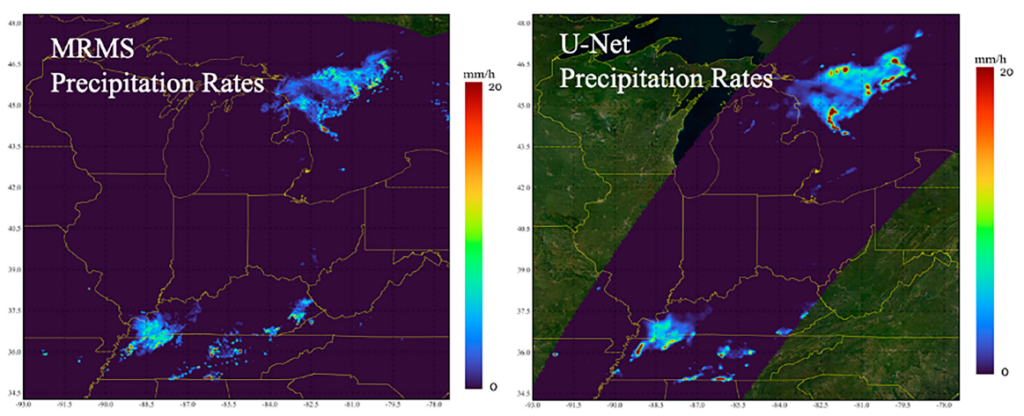
To access the paper, click here: “Optimal Summertime Precipitation Data from GEO and LEO Observations”.