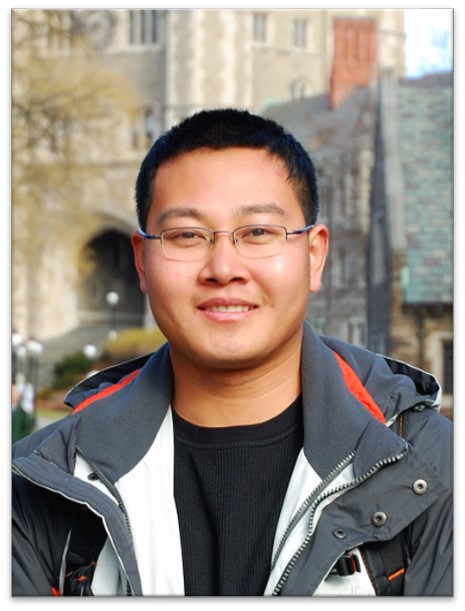
Enhancement of NOAA Snowfall Product Through Machine Learning
This event has passed. See the seminar recording here: Dr. Yongzhen Fan Earth System Science Interdisciplinary Center University of Maryland Monday November 22, 2021, 2
This event has passed. See the seminar recording here: Dr. Yongzhen Fan Earth System Science Interdisciplinary Center University of Maryland Monday November 22, 2021, 2
Last week, NOAA NESDIS held a two-day virtual workshop on “Precipitation Estimation from LEO Satellites: Retrieval and Applications”. The workshop was organized by CISESS Consortium Scientist Kuolin Hsu at University of California, Irvine through a task funded by NESDIS’ Joint Polar Satellite System (JPSS) Program Office. The primary goal of the workshop was to determine future satellite observation requirements for global precipitation. The workshop had nearly 100 participants for each of the four sessions that spanned two days.
The Snowfall Rate (SFR) team at STAR and ESSIC/CISESS, including Huan Meng, Yongzhen Fan, and Jun Dong, has made some notable progress recently that significantly enhances the product and its accessibility to users.
The STAR scientist team of Huan Meng, Yongzhen Fan, Jun Dong, and Yalei You examined the performance of snowfall estimates from the passive microwave snowfall rate (SFR) product for the late season snowstorm that hit Washington and Oregon on April 13. The storm set the local record for most snow accumulation this late in the season, causing power outages and road closures across Portland, Oregon.
The first nor’easter of 2022 swept through the Mid-Atlantic and the Northeast on January 2-4, 2022, resulting in a heavy snow accumulation of up to 14 inches in Virginia and southern Maryland and stranding hundreds of drivers on Interstate 95 in Virginia. The NOAA NESDIS Snowfall Rate (SFR) product captured the evolution of the snowstorm with retrievals from the Advanced Technology Microwave Sounder (ATMS) sensor aboard the S-NPP and NOAA-20 satellite missions, and the AMSU-A/MHS sensors aboard NOAA-19, Metop-B, and Metop-C.
The ESSIC/CISESS snowfall rate (SFR) team, Huan Meng, Jun Dong, and Yongzhen Fan, set up a webpage for the NWS Sterling, VA Weather Forecast Office (Office Call Sign: LWX) at the request of Luis Rosa, a senior forecaster from the office. The page is set for the LWX county warning area (CWA). Currently, the page has the operational SFR images from five satellites but will be expanded to include the experimental SFR from four other satellites. The SFR product is produced at CISESS from direct broadcast data retrieved from the University of Wisconsin. The product latency ranges from 12-25 min depending on the satellite.
ESSIC/CISESS scientists Huan Meng and Yongzhen Fan have recently developed a new machine learning snowfall detection (SD) algorithm, based on eXtreme Gradient Boosting (XGB). The algorithm was developed for the Advanced Technology Microwave Sounder (ATMS) onboard NPP and NOAA-20 as well as the MHS/AMSU-A onboard Metop-A, Metop-B, Metop-C and NOAA-19.